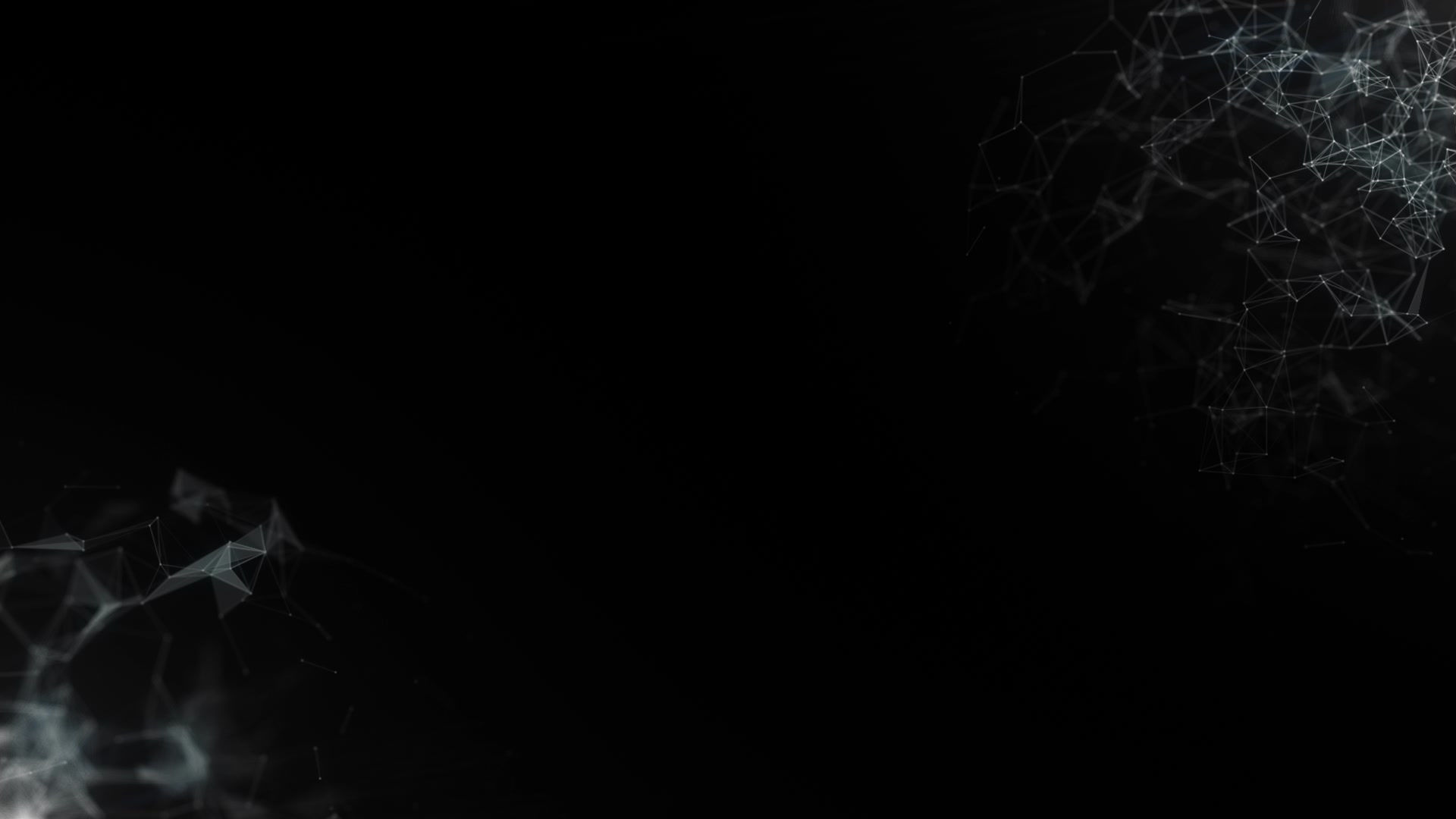
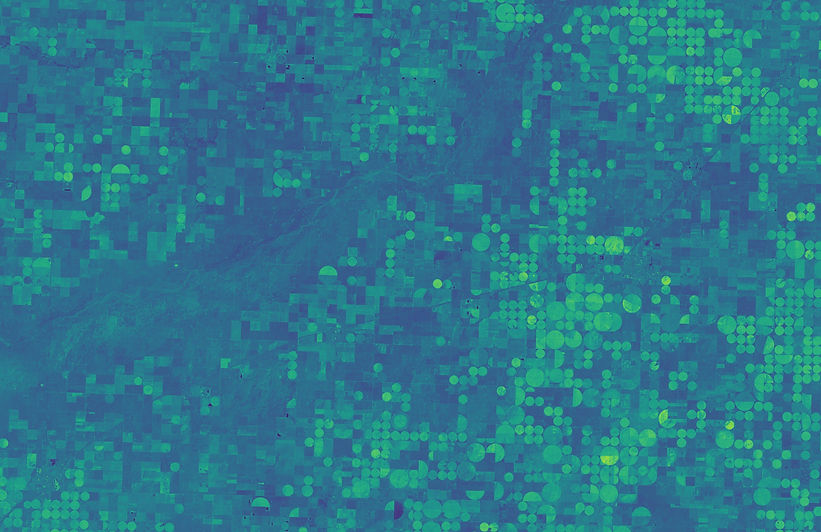
Remote Sensing
Digital Farming
From the sky to the farm
Current research projects in this area:

In recent years, interest in the quality level of soybeans produced in the United States has increased. In this sense, a large proportion of producers claim that they would be willing to invest a greater amount of inputs and technologies in exchange for the benefits received from a higher proportion of oil and protein production in soybeans. For this reason, for some time now, using modern machine learning techniques and remote sensing data, models have been developed in order to spatially estimate the concentration of protein and oil in soybeans at the producer level before harvest. Some findings regarding this were the location of the best moment to make the estimates, which were the best models that worked best and what were the levels of errors in the predictions, having these less than 1.5% of RMSE. This could represent a competitive advantage for those who are seeking to know the quality levels within the soybean fields, helping various actors in the production chain.
On-farm soybean seed protein and oil prediction using satellite data
Carlos Hernandez
Remote Sensing & Digital Farming
Carlos Hernandez, Adrian correndo, Aaron Presthold,
Peter Kyveryga, Ignacio Ciampitti
Fields often show different productivity patterns from year to year. These patterns are mainly caused by changes in the environmental conditions in which they have been immersed. Specifically, differences in precipitations before and during the crop growing season can significantly impact the spatial pattern of productivity, resulting in yield fluctuations and, in turn, interacting with other environmental factors, such as the presence of groundwater. In this sense, a spatial information layer is currently under development using modern techniques of spatial statistics and historical data from remote sensors. The layer, based on an index, illustrates the temporal dynamics of productivity in response to changes in water conditions. In this way, these new datasets could provide innovative insights to decision-makers, identifying which sectors at field level are more unstable and more susceptible to climate variations.
M.S. Student -Emmanuela van Versendaal
Digital Farming
Characterization of temporal field behavior through a high-performance spatial layer that combines remote sensing and spatial statistics
Emmanuela van Versendaal, Carlos Hernandez, Peter Kyveryga ,Ignacio Ciampitti
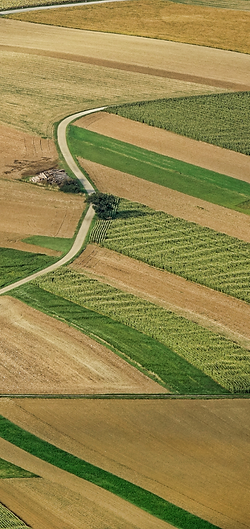
Modern genetic modification techniques can change the growth pattern of roots, affecting the uptake of resources such as water and nutrients. In this sense, changes in the rooting pattern could also change the nitrogen uptake from the soil. Innovative digital phenotyping techniques can help to know with great precision the root system of crops in order to characterize the differences between roots of genotypes with different resource capture capacities. Through the taking of a large number of images using cameras located at different angles, three-dimensional models are being developed that show each part of the roots of different treatments. Using big data techniques and parallel computing, characteristics of corn roots such as length, quantity, type, angle, occupied volume, among others, are being extracted. Through these techniques, it is expected to carry out a high-yield characterization that will serve as a guide in the development and improvement of new maize hybrids aimed at greater efficiency in nutrient capture.
Ph.D. Student - Francisco Palmero
Digital Phenotypying

3D characterization of maize genotype roots through high-performance digital phenotyping using low-cost equipment
Francisco Palmero, Carlos Hernandez, Abhi Pangaladal, Leandro Mas, Benjamin Vail, Edwin Brokesh, Ignacio Ciampitti
Matias Lucero, Carlos Hernandez, Kevin Hamilton, Ignacio Ciampitti
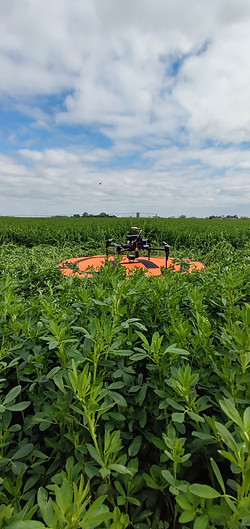
Matias Lucero
Digital Farming
Alfalfa biomass and quality prediction using machine learning and remote sensing data
Satellite images are a useful tool in decision-making support to optimize the management practices at the on-farm level. Based on, estimating pasture growth to determine the better cutting moment to maximize yield in biomass without compromising the quality parameters such as crude protein and fiber. In this case, working with alfalfa (Medicago sativa L.) which is one of the most important crops in the world and in the United States. For this purpose, samples were collected in various pasture stages in three different Kansas fields in 2022. In the examples, height was observed and collected biomass. As a goal, models based on satellite images with a predictive focus on biomass and the quality of alfalfa are in development. The use of geospatial data analytics together with remote sensing could scale and help farmers to improve and optimize the alfalfa crop production.
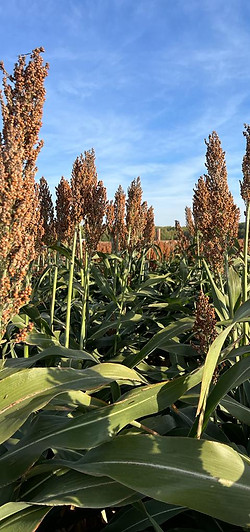
Deep learning methods using imagery from a smartphone for recognizing sorghum panicles and counting grains at an on-farm scale
MS Student - Gustavo Nocera Santiago
Digital Farming
Location: Wamego, KS.
Sorghum Checkoff and Corteva Agriscience for providing support for field trials, investigation, data collection, and labeling process of sorghum imagery. Contribution no. 24-XYZ-J from the Kansas Agricultural Experiment Station.
This study was proposed considering the labor required to estimate field trait characterization and yield gains for sorghum crops from panicles at the field scale. To overcome this bottleneck, we i) detected and segmented sorghum panicles, ii) counted the number of grains within the segmented panicle, and iii) from the counting, estimated the number of grains of the whole panicle. The images used in this study were obtained from smartphone imagery at an on-farm scale. We used and developed machine learning and linear models to achieve our objective, having an average error of 17%.
Location: Kansas and Oklahoma
Project funded by USDA-NRCS
MS Student - Carlos Hernandez
Digital Farming
Quantifying Nutrient Budgets in the Farmer-to-Farmer Digital Conservation Network (F2F_DCN).
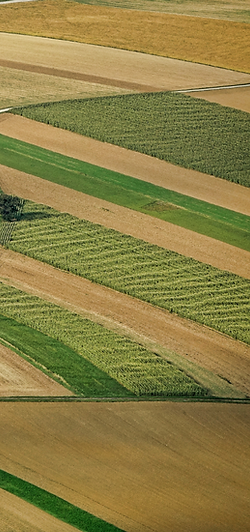
The primary goals of launching the Farmer-to-Farmer Digital Conservation Network (F2F_DCN) project are (i) to establish a network of collaborators that generates nutrient budget information at the farm level that serve to update and integrate technologies for standards of NRCS, and (ii) to provide stakeholders’ education programs concerning the implementation of data management technologies at the farmer level. Our on-farm collaborations will be rooted in farmer-run research; thus, integrated information will be produced from the network and active feedback to the farmers. With a special focus on nitrogen (N), the team will aim to obtain new information on nutrient budgets that are accurate and practical at the field-scale to explore innovative conservation practices. A team of experts on farming systems, soil fertility and crop nutrition, remote sensing, socioeconomics, industry partners, and farmers will join efforts to empowering farmers across Kansas and Oklahoma to obtain accurate and cost-effective nutrient budget estimates
Previous Projects in this area:
The coastal polders of Bangladesh are a critical component of the country's food security, with rice (Oryza sativa L.) being the predominant crop grown in this region. These fields are characterized by a combination of traditional and modern rice varieties, with the latter often yielding almost twice as much as the former. However, the adoption of modern varieties in the polder areas has been limited, mainly due to insufficient data on the rate and extent of adoption. This project aimed to fill this gap by developing a rice variety mapping and adoption methodology that will be useful for policy implications. Our approach allowed the identification of short-term shifts and fluctuations in the rate of adoption of modern rice varieties from the 2016-17 to 2022-23 wet seasons. Future research should aim to extend this approach to other crops and to include the cropping system, thereby allowing the assessment of relevant changes in crop rotation over time. The creation and regular updating of regional and polder-specific crop maps can more effectively support the implementation of interventions and new policies to improve food security in this vulnerable region.
Post Doctoral Researcher - Danilo Tedesco
Remote Sensing
Mapping and Evaluating Rice Variety Adoption in Bangladesh's Polders Using Time-Series Remote Sensing
Danilo Tedesco, Carlos Hernandez, Ana J.P. Carcedo, Luciana Nieto, P.V. Vara Prasad, Manoranjan K. Mondal, Ashish Sutradhar, Jayanta Bhattacharya, Mary Ann A. Batas, Krishna Jagadish, Sudhir Yadav, Ignacio Ciampitti.
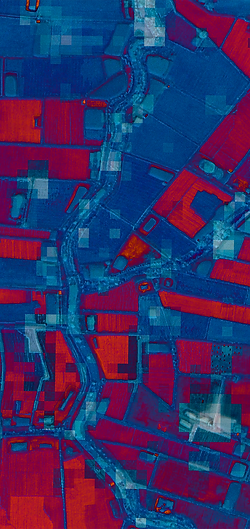
Nitrogen (N) management in corn (Zea mays L.) based on soil diagnosis requires monitoring to readjust the rate applied at sowing. This work aimed to evaluate N monitoring in corn from remote optical sensors and Synthetic Aperture Radar. Eleven N fertilization trials were conducted in commercial corn fields in the southeastern and northern Pampean Region, Argentina. The results showed that monitoring aerial biomass, N concentration and accumulation, N nutrition index (NNI), and soil moisture is possible. The relationship between NNI and yield relative to vegetative periods improved when the N status of the crop was integrated with water status. This information is elementary to re-fertilization in real corn production systems.
Adrian Lapaz
Remote Sensing
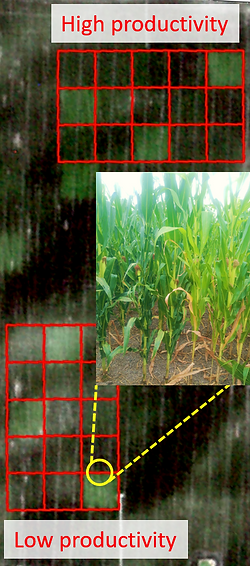.png)
Adrian Lapaz Olveira, Hernan Sainz Rozas, Mauricio Castro-Franco, Monica Balzarini, Walter Carciochi, Adrián Correndo, Carlos Hernandez, Luciana Nieto, Josefina Lacasa, Ignacio Ciampitti, Nahuel Reussi Calvo
Spatio-temporal estimation of nitrogen availability in corn using remote optical sensors and Synthetic Aperture Radar
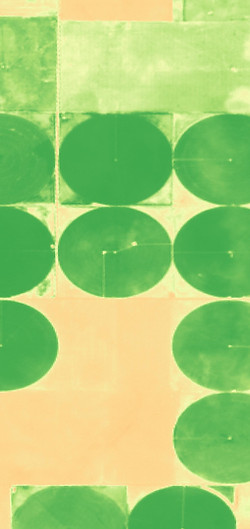
Improving accuracy to assess crop phenology and their space-time fluctuations are essential for farmers, policy makers, and government agencies. Weekly field survey data from extension agricultural agents is currently utilized to generate reports on progress estimates of crop phenology. Although this data is critical, it is labor-intensive, time-consuming, and prone to human error. Remote sensing can improve the accuracy of these estimates via reducing bias and missing data, but this process requires gathering high observational frequency and spatial resolution, combining multi-sensor data streams. Robust classifiers (e.g., random forest, RF) are also a key component for handling large datasets with acceptable trade-off and stability. The aim of this study focuses on comparing the output of a RF classifier model (dealing with imbalanced datasets) testing two different satellite sources (Planet Fusion; PF and Sentinel-2; S-2) in combination with weather data to improve maize ( Zea mays L.) crop phenology classification evaluated in two regions (Southwest and Central) in Kansas (US) during the 2017 season. Our findings showed that the use of very high temporal resolution data (PF) resulted in higher classification metrics (f1-score = 0.94) in two contrasting maize producing regions when compared to S-2 (f1-score = 0.86). In addition, testing the ability of using S-2 to expand the temporal prediction of crop phenology, we observed a drop in f1-score with values of 0.74 and 0.60. This research establishes the value of having access to very high temporal resolution (daily basis) for crop monitoring and delivering actionable insights to the agricultural decision-making process.
For more information, visit Luciana's at:
Enhanced temporal resolution of satellite imagery can assist to improve maize crop phenology classification
Dr. Rasmus Houborg, Dr. Ariel Zajdband, Dr. Arin Jumpasut,
Dr. P. V. Vara Prasad, Dr. Bradley J.S.C. Olson , Dr. Ignacio A. Ciampitti
Ph.D. Candidate - Luciana Nieto
Remote Sensing
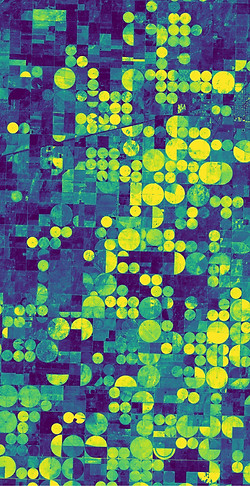
Efficient and more accurate reporting of maize (Zea mays L.) phenology, crop condition, and progress is crucial for agronomists and policymakers. Integration of remote sensing (satellite imagery) with machine learning models has shown a great potential to improve crop classification and provide an in-season progress report for field crops. For this research we focused on integrating ground-truthing field data of maize crop phenology, satellite imagery (Landsat 8), and weather data in order to achieve the following objectives:
1) model training and validation – find the best combination of spectral bands, vegetation indices (VIs), weather parameters, geolocation, and ground truth data, leading to a model with the highest accuracy across years at each season segment (step one);
2) model testing – once the model has been selected, test the performance for each phenology class using unseen data (hold-out cross-validation) (step two).
Dr. Raí Schwalbert, Dr. P. V. Vara Prasad, Dr. Bradley J.S.C. Olson , Dr. Ignacio A. Ciampitti
Towards a scalable satellite-based model for monitoring seasonal maize phenology
Ph.D. Candidate - Luciana Nieto
Remote Sensing
Project funded by Kansas Corn Commission and Crop Quest inc.